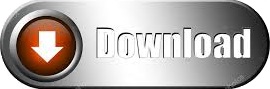
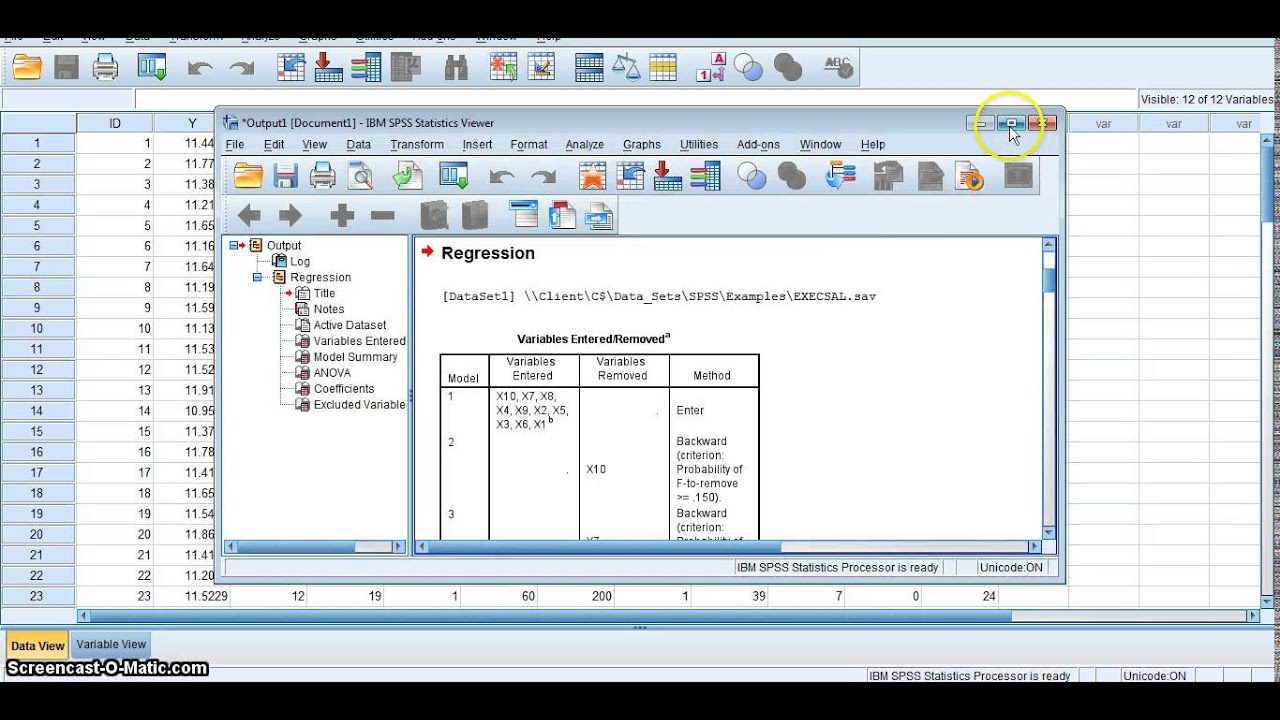
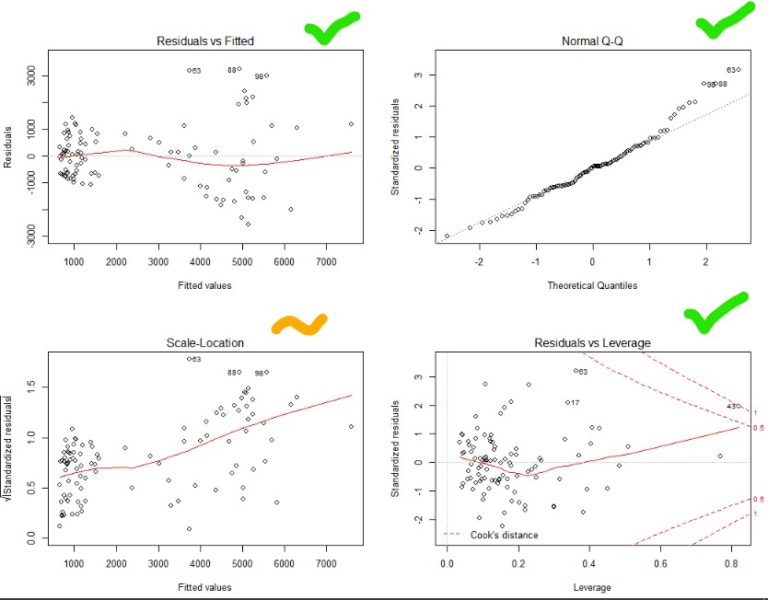
It looks as if the strongest relationship exists between either y and x 2 or between y and x 4 - and therefore, perhaps either x 2 or x 4 should enter the stepwise model first. You can get a hunch of which predictors are good candidates for being the first to enter the stepwise model. Now, if you study the scatter plot matrix of the data: Predictor x 3: % of tetracalcium alumino ferrite.
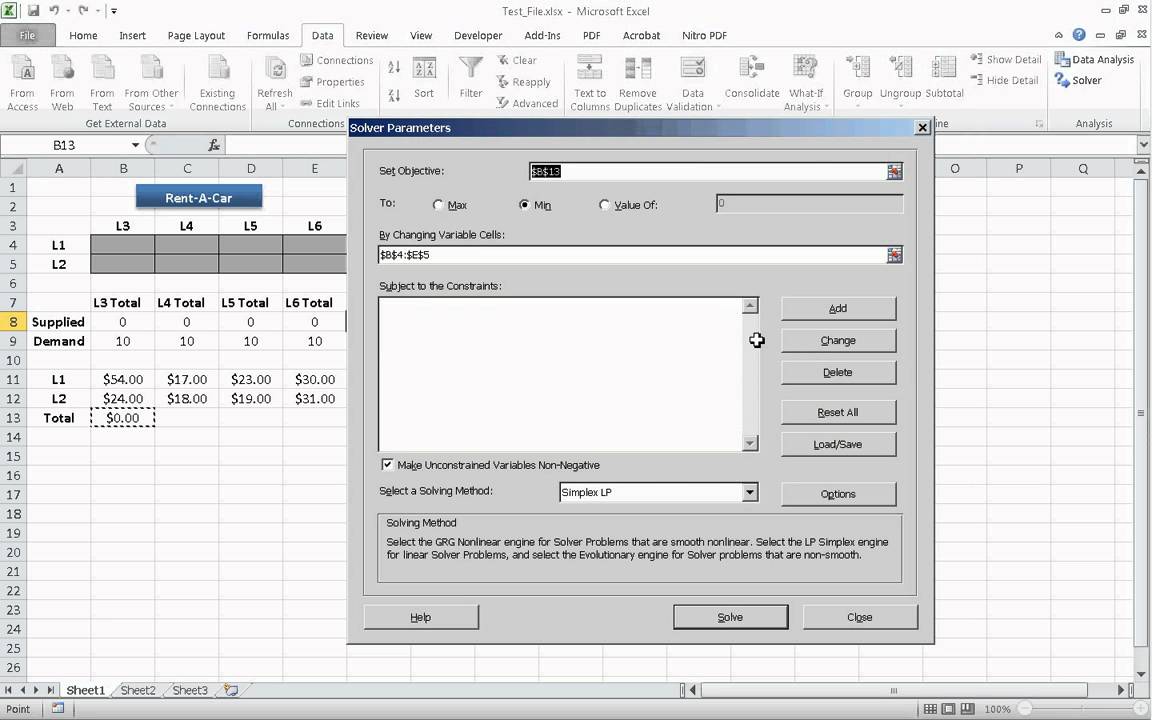
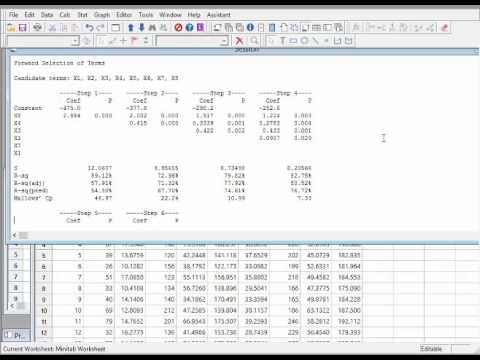
Otherwise, we are sure to end up with a regression model that is underspecified and therefore misleading. This leads us to a fundamental rule of the stepwise regression procedure - the list of candidate predictor variables must include all of the variables that actually predict the response. There is one sure way of ending up with a model that is certain to be underspecified - and that's if the set of candidate predictor variables doesn't include all of the variables that actually predict the response. Our hope is, of course, that we end up with a reasonable and useful regression model. While we will soon learn the finer details, the general idea behind the stepwise regression procedure is that we build our regression model from a set of candidate predictor variables by entering and removing predictors - in a stepwise manner - into our model until there is no justifiable reason to enter or remove any more. In this section, we learn about the stepwise regression procedure.
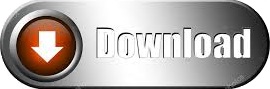